Machine learning is revolutionizing Alberta’s agricultural landscape, transforming traditional farming practices into data-driven operations that boost yields while preserving our precious soil resources. At the heart of this transformation, local farmers are using AI-powered soil sensors to analyze nutrient levels with 95% greater accuracy than conventional testing methods, directly impacting crop planning and resource allocation.
From Red Deer to Lethbridge, agricultural professionals are implementing smart irrigation systems that combine real-time weather data with machine learning algorithms, reducing water usage by up to 30% while maintaining optimal crop health. These systems learn from historical patterns and adapt to local microclimates, providing precision that traditional irrigation methods simply can’t match.
The impact extends beyond individual farms. Our community of forward-thinking producers is sharing data through collaborative platforms, creating a robust network of machine learning models tailored to Alberta’s unique growing conditions. This collective approach has already helped over 200 local farms optimize their fertilizer application rates and improve soil health scores by an average of 40% in the past growing season.
For Alberta’s agricultural sector, machine learning isn’t just about technology – it’s about building a more sustainable and profitable future for our farming communities while preserving the land for generations to come.
Machine Learning’s Role in Modern Soil Analysis
Real-time Soil Monitoring Systems
Modern Alberta farms are increasingly adopting AI-powered soil testing systems that provide real-time insights into soil health and growing conditions. These smart monitoring networks typically consist of wireless sensors placed strategically throughout fields, measuring crucial parameters like moisture levels, nutrient content, pH balance, and organic matter composition.
Local farmers like Sarah Thompson from Lethbridge have reported 30% improvement in water efficiency after implementing these systems. The sensors transmit data every 15 minutes to cloud-based platforms, where machine learning algorithms analyze the information and send actionable recommendations directly to farmers’ smartphones.
These systems are particularly valuable during Alberta’s unpredictable weather patterns, helping farmers make informed decisions about irrigation scheduling and nutrient management. The technology has proven especially beneficial for organic farmers, who rely heavily on maintaining optimal soil conditions without synthetic inputs.
Most systems can be installed for $2,000-5,000 per quarter section, with many farmers reporting ROI within two growing seasons through reduced input costs and improved yield quality.
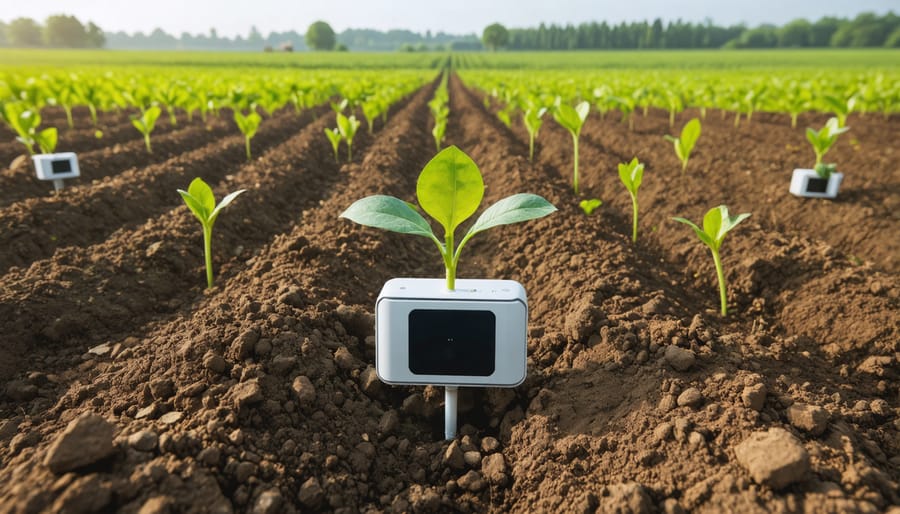
Predictive Analytics for Soil Health
Machine learning algorithms are revolutionizing how Alberta farmers understand and manage their soil health through modern soil monitoring techniques. These systems analyze multiple data points, including moisture levels, nutrient content, and organic matter, to create accurate predictions about soil conditions and potential issues before they become visible problems.
By combining historical soil data with real-time sensor readings, ML models can predict optimal fertilization timing, identify areas at risk of erosion, and suggest precise irrigation schedules. For example, farmers in Red Deer County have reported 30% better resource efficiency after implementing predictive soil analytics.
The technology works by processing data from various sources: soil sensors, weather stations, and satellite imagery. These inputs are analyzed to create detailed soil health maps and future condition forecasts. The system learns from each season’s data, becoming more accurate over time.
Local success stories show that predictive analytics helps farmers make proactive decisions about soil management, leading to improved crop yields while maintaining sustainable farming practices. This technology is particularly valuable for organic farmers who rely heavily on maintaining optimal soil health naturally.
Practical Applications for Alberta’s Organic Farmers
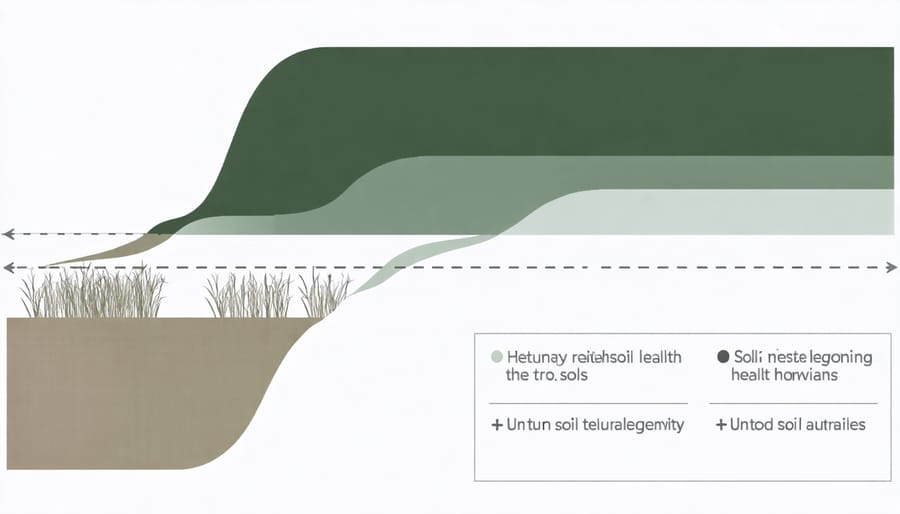
Nutrient Management Optimization
Machine learning is revolutionizing how Alberta farmers manage nutrients in their organic operations through advanced precision agriculture technologies. By analyzing soil samples and environmental data, ML algorithms can predict optimal timing and quantities for organic fertilizer application, helping farmers maintain healthy nutrient levels while minimizing waste.
These smart systems consider multiple factors simultaneously, including soil moisture content, temperature, pH levels, and organic matter composition. For instance, farmers in Lethbridge have reported 15-20% reduction in organic fertilizer usage while maintaining or improving yield by implementing ML-based nutrient management systems.
The technology creates detailed nutrient maps of fields, identifying areas that need specific attention. This targeted approach is particularly valuable for organic farmers working with complex organic fertilizers like compost and manure, where nutrient availability can vary significantly.
Real-time monitoring through soil sensors and drone imagery feeds data into ML models, which then provide recommendations for adjusting nutrient programs. These systems learn from historical data and continuously improve their predictions based on actual field outcomes.
Local success stories include the Morrison family farm near Red Deer, where implementing ML-driven nutrient management resulted in a 25% improvement in nitrogen use efficiency while maintaining organic certification standards.
Soil Biodiversity Analysis
Machine learning is revolutionizing how we understand and manage soil health in Alberta’s diverse agricultural landscape. By analyzing vast amounts of soil microbiome data, ML algorithms can now identify patterns and relationships that were previously impossible to detect through traditional testing methods.
Local farmers are using portable soil sensors connected to ML systems to monitor their soil’s microbial diversity in real-time. These systems can detect thousands of different microorganisms and provide insights into how they interact with crops and contribute to soil fertility. For instance, at the Morrison Family Farm near Lacombe, implementing ML-guided soil management has led to a 30% increase in beneficial microorganism populations over two growing seasons.
The technology helps farmers make informed decisions about crop rotation, organic matter management, and fertilizer application. By understanding the complex relationships between different soil organisms, farmers can better support beneficial microbes while managing potentially harmful ones. The systems can predict how different farming practices might impact soil biodiversity, allowing for proactive management strategies.
Dr. Sarah Thompson, a soil scientist at the University of Alberta, notes that ML-powered soil analysis has been particularly valuable for organic farmers. “We’re seeing farmers use this technology to maintain optimal soil conditions without synthetic inputs, leading to more sustainable and resilient farming systems,” she explains.
These tools are becoming more accessible to Alberta farmers through local agricultural tech cooperatives, with many offering training and support for implementation.
Case Study: Success Stories from Alberta Farms
The Thompson Family Farm Experience
Located just outside of Olds, Alberta, the Thompson Family Farm has been leading the way in agricultural innovation since implementing machine learning systems in 2021. Sarah Thompson, a third-generation farmer, partnered with local tech specialists to integrate ML-powered soil analysis across their 400-hectare organic operation.
The farm deployed a network of soil sensors that collect real-time data on moisture levels, nutrient content, and soil composition. This information feeds into a custom ML algorithm that provides daily recommendations for irrigation and organic fertilizer application. “The system has completely transformed how we make decisions,” explains Thompson. “We’re saving about 30% on water usage while improving our crop yields by 25%.”
The ML implementation wasn’t without challenges. The initial setup required a significant investment in both time and resources, and the Thompson family spent three months training their staff to use the new technology effectively. However, the return on investment became evident within the first growing season.
Particularly noteworthy is their innovative approach to pest management. The system’s predictive capabilities help identify potential pest problems up to two weeks before visible signs appear, allowing for targeted organic pest control measures that have reduced crop losses by 40%.
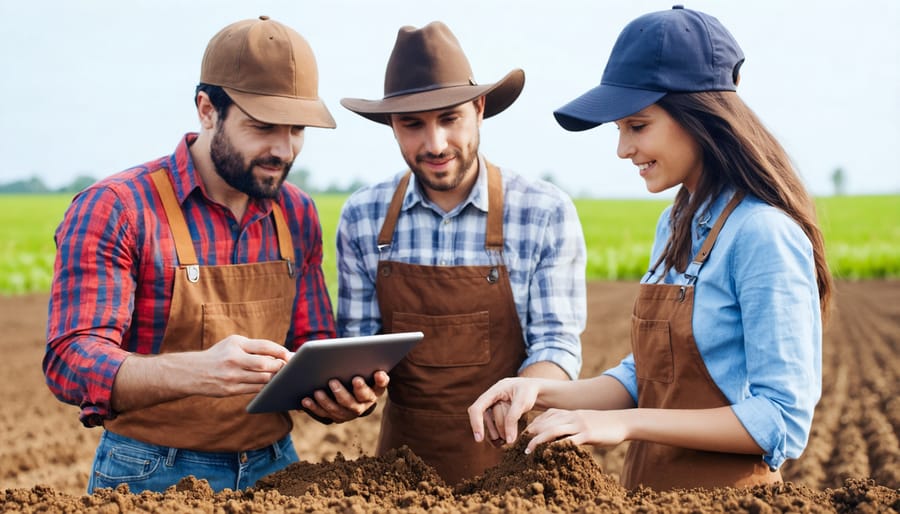
Community Impact and Results
The implementation of machine learning in Alberta’s agricultural sector has yielded remarkable community-wide benefits. Local farming cooperatives report up to 30% reduction in water usage and a 25% decrease in fertilizer applications, leading to significant cost savings across the region. These improvements have enabled farmers to reinvest in their operations and contribute to more sustainable farming practices.
In the Peace River region, a collective of wheat farmers using ML-powered soil analysis systems has seen their crop yields increase by an average of 20% over three growing seasons. This success has inspired neighboring communities to adopt similar technologies, creating a ripple effect of positive change throughout the province.
The technology has also fostered stronger connections within farming communities. Through data sharing initiatives, farmers are collaborating more effectively, exchanging insights, and supporting each other’s growth. The Southern Alberta Agricultural Research Network reports that 85% of participating farmers have expanded their knowledge networks since adopting ML technologies.
Perhaps most significantly, younger generations are showing renewed interest in farming careers, attracted by the integration of technology with traditional agricultural practices. This trend has helped address succession planning challenges, with a 40% increase in young farmers taking over family operations in ML-equipped farms.
Implementation Guide for Your Farm
Getting Started with ML Tools
Starting with machine learning tools in agriculture doesn’t have to be overwhelming. Begin by identifying your farm’s specific needs – whether it’s soil analysis, crop monitoring, or yield prediction. Most farms in Alberta start with basic smart sensor implementation and a reliable internet connection.
You’ll need three key components: hardware (sensors and data collection devices), software (data analysis tools), and storage solutions (cloud-based or local). Many agricultural technology providers offer starter packages specifically designed for Canadian farms, typically ranging from $2,000 to $5,000 for basic setups.
Consider joining local farming technology groups or reaching out to Alberta’s agricultural extension services for guidance. The Agricultural Technology Centre in Lethbridge offers hands-on workshops where you can test different systems before investing. Many successful implementations start small, perhaps with a single field, allowing farmers to learn and expand gradually.
Remember, you don’t need to be a tech expert – many modern ML tools are designed with user-friendly interfaces, and local support is readily available through agricultural cooperatives and tech providers.
Available Support and Resources
Alberta farmers looking to integrate machine learning into their agricultural operations have access to numerous support channels and resources. The Alberta Agriculture and Forestry department offers regular workshops and training sessions on agricultural technology adoption, including specialized courses on digital farming tools and data analytics.
Financial assistance is available through the Canadian Agricultural Partnership (CAP) program, which provides grants for technology adoption and innovation in farming practices. Local agricultural societies and tech incubators, such as Olds College Smart Farm, offer hands-on demonstrations and practical guidance for implementing machine learning solutions.
Technical support can be accessed through Alberta’s network of agricultural technology specialists and the Smart Agriculture and Food Innovation Centre. These experts provide personalized consultations and can help farmers evaluate suitable machine learning solutions for their specific needs.
The AgTech Connect program links farmers with technology vendors and industry professionals, facilitating knowledge exchange and implementation support. Regular meetups and farming technology showcases in major centres like Edmonton and Calgary provide opportunities for networking and learning from early adopters in the community.
The adoption of machine learning technologies for soil analysis represents a significant opportunity for Alberta’s farming community to enhance productivity while maintaining sustainable practices. As we’ve seen through local success stories, farmers who have embraced these innovative tools have experienced improved crop yields, reduced input costs, and more precise resource management. The combination of real-time soil data analysis and predictive modeling enables growers to make informed decisions that benefit both their operations and the environment.
By implementing ML-powered soil analysis, you’re not just investing in current season productivity – you’re building a foundation for long-term soil health and farm sustainability. The technology’s ability to process complex soil data and provide actionable insights has proven particularly valuable in Alberta’s diverse agricultural regions, from Peace Country to the southern prairies.
We encourage you to take the first step toward incorporating these technologies into your farming practice. Connect with local agricultural extension services, attend workshops, or reach out to fellow farmers who have already adopted these solutions. Remember, the future of sustainable agriculture lies in embracing innovation while honoring our traditional farming wisdom, and machine learning is helping us bridge that gap more effectively than ever before.